Explore our expert-made templates & start with the right one for you.
Your Log Analytics Architecture Should be a Data Lake
Log analytics is a problem that almost every modern business needs to solve. Whether it’s from remote devices, cloud servers, or your own products, you’re probably collecting a large volume of log data somewhere; and if you’re like most companies, you’re also struggling to derive insights from it in a scalable and cost-effective manner.
This article examines the common architectural challenges of operationalizing log data at scale, and suggests a solution centered around a cloud data lake built and managed using Upsolver on Amazon S3.
What is Log Analytics? Defining the Problem
Log analytics is the process of analyzing machine-generated data to gain operational insights. This data can be obtained from various sources, such as computer networks, mobile devices, IT systems, or web user tracking.
Organizations might use log analytics to:
- Reveal patterns in the way users interact with an app or website.
- Identify problems, audit security activities, and ensure compliance with established rules.
- Forecast demand for IT infrastructure and plan ahead for surges or expected changes.
There are four main challenges when it comes to log analytics: volume, variety, parsing, and speed. Log volume refers to the sheer amount of data that is generated by machine sources, which can make it difficult to manage and monitor. Variety refers to the different formats that logs can come in and lack of a consistent schema, which can make it difficult to standardize and analyze the data. Parsing refers to the process of extracting information from logs, which can be time-consuming and require manual administration. Speed refers to the balance between speed and accuracy when it comes to log analysis, where one often comes at the expense of the other.
These challenges are exacerbated by the nature of the data. Events logs are the archetypical source of streaming data – a continuously-generated stream of semi-structured data, reflecting the state of a software or hardware system at any given moment. Some of the most common scenarios are IoT sensors, server and network security logs, app activity, and advertising data.
While in the past the task of collecting and analyzing logs was associated mainly with IT and networking professionals, the rise of software development, clickstream analysis, and app analytics has led to increased demand for log data analysis by data analytics teams, business units, and other non-IT stakeholders.
Limitations of Databases and SQL
Traditionally, log analysis was seen as another analytical workload that could be offloaded to a database, against which data consumers could use SQL and BI tools to leverage data and generate insights. Elasticsearch, which was released just over a decade ago, has become the de-facto standard in this regard due to its ability to provide strong out-of-the-box performance for search and analytics.
However, as log data grows in volume and velocity, and with increasing requirements to use this data for new types of analysis – dashboards, operational analytics, ML model training, and more – the drawbacks of a database approach become more apparent. These include:
- Cost. Databases lack elasticity, making them expensive and cumbersome to scale due to the need to constantly spin up, configure, and resize clusters based on changes in data volume and retention.
- Latency. Data isn’t available in real-time due to the latencies built in to batch ETL processes.
- Machine learning roadblocks. The database, which was built for OLAP analytics, isn’t a good fit for data scientists, who build models and train them against custom datasets. ML datasets require data from various points in time, making them easier to extract from an append-only log, as opposed to a datastore that allows updates and deletes. Additionally, data scientists want to use scripting in Python/Scala to build their datasets and apply functionality which would be very cumbersome to do in SQL.
- Lock-in. Databases rely on a proprietary data format optimized for their own query engine. This limitation creates vendor lock-in and forces organizations to choose between two bad options: force a use case on an existing database or replicate the data to another store which introduces consistency and reliability issues.
Elasticsearch specifically is a great log analysis and search tool, but it can be expensive to store all of your data in it, especially if you’re working in the cloud. This is because database storage is expensive, and you need to store more than just the logs themselves (replicas, indices, free space, and so on). For example, storing 1 TB of log data in Elasticsearch on AWS could cost $1500/month.
A Decoupled Architecture Solves Some Problems, Creates New Ones
Due to all of the issues above, the database fell out of favor as the core datastore for log data as organizations began searching for more scalable, cost-effective, and agile solutions. Hence, in recent years we have seen the rise of the decoupled architecture, wherein raw data is ingested and stored on inexpensive object storage, with compute resources provided ad-hoc to different analytic services per use case.
This data lake approach bypasses the roadblocks of traditional databases above by storing data as an append-only log on cloud storage, while enabling consumers to analyze the data with best-of-breed engines per use case. With this architecture, scaling is elastic, cost is low, machine learning is natively supported and data is stored in its raw format without ETL delays. This architecture heavily relies on open file formats (such as Apache Parquet) for storage and metadata stores for data discovery and queries.
Logging databases would often still be used, but at much lower cost due to the ability to offload much of the data onto inexpensive object storage. Here is what that process looks like in Upsolver:
Despite these benefits, a data lake is still missing something – namely, all the advantages of a database! Object storage is often messy, complex, and slow to show value, with the main reasons including:
- Data stored on cloud storage can be compared to a bunch of files on disk. Databases were invented precisely to give users easier access to data by letting them run SQL instead of querying files directly, and this functionality is lacking in data lakes.
- On cloud storage, there is no schema or statistics to guide consumers to insights. Instead, data discovery requires manual ETL efforts to pull samples of the data.
- Query latency can be 1000X slower compared to databases that include indexing, an optimized file system, and fast access to disk (much faster than the latency you get with cloud storage).
Addressing these data preparation and management challenges forces companies to spend hundreds of engineering hours on operations such as compacting small files, which are code- and effort-intensive but do not deliver direct value from a business point of view.
Closing the Gap Between Data Lake and Data Consumers
Up to this point we’ve explained why companies want to analyze logs and the challenges they encounter when trying to do this at high scale: databases are fragile and expensive, data lake architectures are complex and effort-intensive.
The significant gap between the data lake and its consumers created the need for a SQL pipeline platform that would operationalize data in cloud object stores. Companies can’t compromise on parameters such as elasticity or readiness for machine learning, so they seek a platform to preserve the functional advantages of databases. This data pipeline platform should offer simple APIs for empowering data consumers and automation for reducing IT overhead.
Query performance and data quality are dependent on companies’ ability to implement a data platform effectively. However, this is often easier said than done. Today, companies use a variety of cloud services and open source projects to build their own data pipelines.
The end result is often closer to the vision of self-service, democratized analytics, but the data-to-insight process is still coding- and IT-intensive, with over 80% of the time spent on building, orchestrating, and scaling data pipelines. Services like AWS EMR or AWS Glue help with the IT overhead, but the technical barrier to entry is still higher compared to writing SQL.
As the big data landscape matures, three types of approaches have emerged:
- Templates. AWS promotes a best-of-breed approach by releasing a service per technical need, and there are already 100s of services. To make it easier for customers to build solutions from multiple services, AWS released Data Lake Formation, which utilizes pre-defined templates to save some of the time spent on writing code and integrating services.
- Databases that utilize a decoupled architecture. Google BigQuery, Snowflake, and DataBricks Delta are data warehouse services that decouple compute from storage. They scale elastically and are priced by consumption of compute and query resources. This is definitely a big improvement compared to traditional RDBMS but the issue of vendor lock-in remains – these services use their own proprietary formats for storage so data can only be read from their query engine, while introducing new analytical engines requires replication of the data to a new store.
- Self-service platforms over data lakes. When building a long-term strategy, companies want to use data lakes for their cost-effectiveness while data consumption tools come and go (later this approach has evolved into what is known as data mesh). To realize this vision, companies need open storage and metadata layers and a platform to keep their chaos organized and optimized. For example, Upsolver is a service located between the AWS data storage layer (S3+Glue Data Catalog) and analytical services such as Athena, Redshift, and RDS. Upsolver enables companies to use a visual interface to operationalize an AWS data lake so it will be accessible and useful for all data consumers. Upsolver does this by adding to Amazon S3 such capabilities as schema discovery, indexing, self-serve data preparation, and performance optimizations.
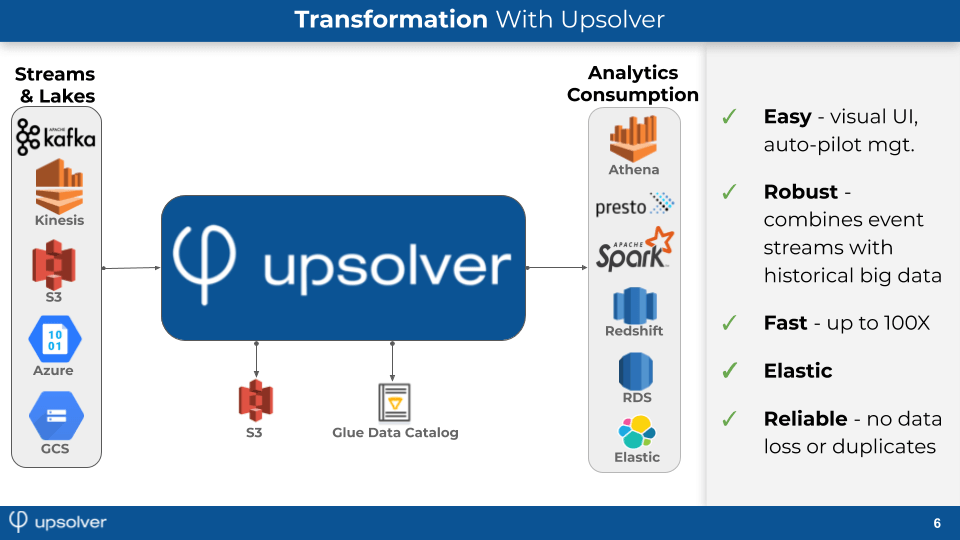
Companies Have a Lot to Gain From Analyzing Their Logs in a High-Performance Data Lake
Log data and event streams play a more prominent part than ever in modern big data architectures. The need to access and operationalize this data for analytics and machine learning is challenging even for massive organizations, and certainly for companies that have limited in-house data engineering resources.
In this blog post, we’ve covered several strategies for taming event log data – from databases to data lakes to modern data platforms. To see value from log data, most companies will have to transition from the former to the latter.
One company that successfully made this transition is Cox Automative. This case study discusses how Cox Automotive uses a data lake to improve operations and standardize security log analytics across its 16 subsidiaries. The company has found that moving its log analytics architecture to an AWS data lake has saved it $700,000 and has allowed it to respond more quickly to security threats.
Next Steps
If you’re ready to implement a next-generation architecture for log analytics, streaming analytics, and big data processing, you could be a good fit for Upsolver. Schedule a call today.
Try SQLake for free (early access). SQLake is Upsolver’s newest offering. It lets you build and run reliable data pipelines on streaming and batch data via an all-SQL experience. Try it for free. No credit card required.